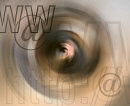
By Rich Garling January 2018
Abstract
There are huge mounds of data being gathered today by a multitude of organizations around the world. Governments, private and public companies, not-for-profit organizations are all gathering data. Over 2.5 quintillion bytes of data are generated and stored per day. The question does arise as to what to do with all that data? Can it serve a useful purpose? Tools for analyzing reams of data of information at speeds and accuracy inconceivable ten years, or even twenty years ago, have been developed. From this data company’s feel, they can derive patterns that will help to increase sales. From this processing, people can determine the proper course of exercise and diet that best fits them. This paper aims to explore the various efforts being used to analyze big data and the rewards and failures that have resulted from this effort.
Introduction
There are huge mounds of data being gathered today by a multitude of organizations around the world. From Governments to private and public companies it is estimated that over 2.5 quintillion bytes of data are generated and stored per day (Laudon, 2016). The question does arise as to what to do with all that data? Why is it being generated? Is there information within this huge mound of data that could be culled for some useful purpose? Many companies and organizations are working toward developing tools that will allow exploring this information at speeds and accuracy unimaginable ten years, or even twenty years ago. Much of this ability to accurately cull massive amounts of data has come about due to advancements in technology and data processing that allow for the analysis of data at greater speeds and accuracy. From this data, companies can derive patterns of customer purchasing. From this processing, people can determine the proper course of exercise and diet that best fits them. This paper will explore the various efforts being used to analyze big data and the rewards and failures that have resulted from this effort.
Types of Big Data Collected
There are many kinds of data gathered from a variety of sources. In many cases companies are gathering data they didn’t realize would have some value, such as addressing customer needs or increasing sales. Green Mountain Coffee had been gathering and storing voice and text data for years. This data went unused until Green Mountain invested in analyzing structured and unstructured audio and text data. Green Mountain uses this analysis to learn more about customer behavior buying habits and patterns. By learning more about what customers want, what issues they were having with their twenty different brands and over two hundred different drinks, Green Mountain could produce information that would lead to increased sales. Information responding to specific points of customer confusion or concern helped to produce answers posted on web pages and social media sites. Customer queries and the answers to those queries became a response used by customer service representatives when responding to similar queries by other customers. All of this analysis led to a better experience for Green Mountain’s customers. AutoZone used data showing the types of automobiles owned by people living near their stores. This data was used to create sales specials unique to that store. AutoZone would use this data to adjust inventory to fit the types of cars prevalent in the neighborhoods surrounding the store.
Technologies Used to Gather Big data
Green Mountain obtained the services of Calabrio Speech Analytics to analyze the mounds of data generated from its call centers. Calabrio provides sophisticated audio and text analytics that unlock the goldmine of information in a contact center, transforming every interaction into usable data (Calabria Speech, 2018). AutoZone (AutoZone, 2018) used NuoDb (NuoDb, 2018) database software system to derive automobile types owned by potential customers surrounding its stores. Sears developed a big data system using Apache Hadoop to target groups within its sixty million credit card customers with special sales and promotions. Sears spent heavily in information technology spending more than all other non-computing firms except for Boeing Corporation. Using Apache Hadoop, Sears was able to analyze immense amounts of data weekly what used to take six weeks using Teradata warehouse software and SAS servers. Sears old system could use only 10% of the data available; today it uses 100% of the data. In the past it could only retain this same data for short periods of time, usually less than ninety days, now it keeps all of the data. Today, Sears sells its knowledge of developing big data analysis tools using Apache Hadoop to other companies by setting up a subsidiary company, Metascale.
Big Data and The Benefits Derived
Sears was at one time the retailer in the United States. Then Wal-Mart, Home Depot, Lowe’s and Amazon came along (D’Onfro, 2015). Sears has been losing ground ever since and was looking for a way to stop the bleeding. Sears realized it had a huge customer base which contained unseen data. Sears determined that it could use this data to help stem the tide and turn around its fortunes. By investing heavily in information technology, Sears figured it could regain ground lost by increasing sales to this huge customer base. With sixty million potential customers, it all made sense. By investing in Apache Hadoop, it could better analyze the data it had and identify targeted groups in which to sell products. A deeper understanding of customer buying habits or patterns would increase sales. Sears has had incomplete success since it has failed to address the fundamental issue of Sears’s cost structure. Sears’s cost structure is amongst the highest in the industry, and it has kept it from translating its big data efforts into success. Green Mountain Coffee wanted to improve the customer experience by addressing points of confusion and their buying habits. Using this information to address customer needs and requirements, it theorized, would help to increase sales and solidify the market position. Today, management can quickly identify pain points and issues before they get out of hand.
Where Big Data Worked
Examples of decisions where big data has helped improve either products or services are prevalent in consumer applications. Personal devices companies, such as Fitbit, Sony, and Garmin have helped people to analyze their exercise routines, diets, and sleep patterns (Laudon, 2016). These devices connect to the internet allowing users to join with others users to compare how their routines are working in comparison to others. Under Armour’s (UA) Map My Walk allows users to create a profile, log workouts from walking, running, and bicycle riding; even over different terrains. UA is a mobile device application commonly used on iPhones or Android devices It tracks users routines, sets up diets for them, creates goal setting, and users can join any number of groups worldwide (UA Record, 2018). Skyscanner and Trivago (Trivago, 2016) use big data systems to provide mobile applications allowing travelers to determine the best options available for purchasing airline tickets, reserving hotel rooms, and renting cars when traveling.
Where Big Data Did Not Work
But not all big data ventures are advisable or well thought out. Google developed an algorithm it claimed could accurately show how many people nationwide had contracted influenza. Google theorized it could determine the number of people with influenza and their locations by using the search data from its search engine. The numbers Google showed constantly over-estimated flu rates when compared to conventional data gathered by other groups including the Center for Disease Control and Prevention. What Google failed to take into account, searches are sometimes controlled by emotion. The number of searches increased as media coverage and social media posts increased, which caused an inflated number of returns in a Google search. Sears’s use of big data has, so far, not brought it back to a profitable state. One theory may be that it is not asking the right questions in which to query their huge amounts of data. Until Sears fixes its broken cost structure, using big data; even selling it to its competitors, will not right this broken ship (Laudon, 2016). Wal-Mart understood that it needed to control its cost structure (Songini, 2006). Sears has yet to grasp it.
Conclusion
In conclusion, does big data bring big rewards? It can if the right questions are asked. Google and Sears are examples of where the right questions are not being asked. Sears was close, but it failed to fix fundamental problems with its structure, it was unable to put itself in a position of competitive advantage. Green Mountain and Starbucks have both utilized big data to meet customer needs (Huff, 2014). AutoZone can control its inventory to meet customer needs and control costs. Travelers now enjoy the ability to change travel on the fly. Amazon allows its customer to do comparison shopping with competitors selling similar or same products even if they’re not on Amazon (D’Onfro, 2015) (Peterson, 2015). Big Data analysis has its benefits, but it has drawbacks. Much is dependent on asking the right questions.
References:
AutoZone | Auto Parts & Accessories | Repair Guides & More. (n.d.).
Retrieved January 28, 2018, from https://www.autozone.com/
D’Onfro, J. (2015, July 25). Wal-Mart is losing the war against Amazon. Retrieved from
http://www.businessinsider.com/wal-mart-ecommerce-vs-amazon-2015-7
Huff, T. (2014, August 23). How Starbucks Crushes It on Social Media | Social Media Today. Retrieved from
http://www.socialmediatoday.com/content/how-starbucks-crushes-it-social-media
Laudon, K. C., & Laudon, J. P. (2016). Management information systems: Managing the
digital firm (14th ed.). Boston, MA: Pearson Education, Inc.
NuoDB. (n.d.). Retrieved January 28, 2018, from https://www.nuodb.com/
Peterson, H. (2015, July 13). The key differences between Wal-Mart and Amazon in
one chart. Retrieved from
http://www.businessinsider.com/amazon-vs-wal-mart-in-one-chart-2015-7
Songini, M. (2006, March 2). Wal-Mart details its RFID journey | Computerworld.
Retrieved from http://www.computerworld.com/article/2562768/enterprise-resource-planning/wal-mart-details-its-rfid-journey.html
Speech, Text & Desktop Analytics for the Contact Center | Calabrio ONE. (2018,
January 28). Retrieved January 28, 2018, from https://www.calabrio.com/products/calabrio-analytics/trivago.com – The world’s top hotel price comparison site. (n.d.). Retrieved January 28, 2018,
from https://www.trivago.com/
UA Record? Health & Fitness Network | Under Armour. (n.d.). Retrieved January 28, 2018, from https://www.underarmour.com/en-us/ua-record?iid=bucket